
What is Data Analytics | Full Information|
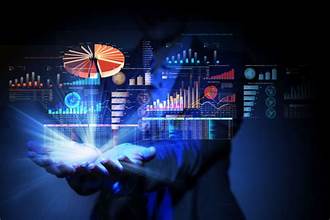
Data analytics is the process of examining large and varied data sets to uncover hidden patterns, correlations, trends, and insights. It involves using various techniques, tools, and methodologies to transform raw data into actionable information for decision-making and strategic planning. Data analytics encompasses a wide range of approaches, from simple descriptive statistics to advanced predictive and prescriptive modeling. Here’s a comprehensive overview of data analytics:
- Types of Data Analytics:
- Descriptive Analytics: Descriptive analytics involves summarizing historical data to gain insights into past performance and understand what has happened. It includes techniques such as data visualization, summary statistics, and exploratory data analysis (EDA).
- Diagnostic Analytics: Diagnostic analytics focuses on identifying the causes of past events or outcomes by analyzing historical data. It aims to answer the question “Why did it happen?” and often involves root cause analysis and hypothesis testing.
- Predictive Analytics: Predictive analytics leverages historical data to forecast future trends, behaviors, or outcomes. It uses statistical modeling, machine learning algorithms, and data mining techniques to make predictions based on patterns observed in the data.
- Prescriptive Analytics: Prescriptive analytics goes beyond predicting future outcomes by recommending actions or decisions to achieve desired objectives. It combines predictive modeling with optimization techniques to provide actionable insights and decision support.
- Key Components of Data Analytics:
- Data Collection: The first step in data analytics involves gathering, acquiring, and collating data from various sources, including databases, files, sensors, and streaming sources.
- Data Preparation: Data preparation involves cleaning, filtering, transforming, and organizing raw data to make it suitable for analysis. This may include data cleaning, missing value imputation, feature engineering, and normalization.
- Data Analysis: Data analysis encompasses the exploration, manipulation, and interpretation of data using statistical and computational techniques. This involves applying various analytical methods to extract insights and derive meaningful conclusions from the data.
- Data Visualization: Data visualization is the graphical representation of data to visually communicate insights, patterns, and trends. Visualization techniques include charts, graphs, heatmaps, and interactive dashboards.
- Modeling and Algorithms: Modeling involves building mathematical or computational models to represent relationships and patterns in the data. This includes regression analysis, machine learning algorithms, time series forecasting, and optimization techniques.
- Applications of Data Analytics:
- Business Intelligence: Data analytics is widely used in business intelligence (BI) to analyze sales data, customer behavior, market trends, and financial performance to support decision-making and strategic planning.
- Marketing and Customer Analytics: Data analytics helps organizations understand customer preferences, segment customers, personalize marketing campaigns, and optimize customer acquisition and retention strategies.
- Healthcare Analytics: In healthcare, data analytics is used for clinical decision support, disease surveillance, patient monitoring, predictive modeling, and healthcare management optimization.
- Finance and Risk Analytics: Data analytics is employed in finance for fraud detection, credit scoring, portfolio management, risk assessment, and algorithmic trading.
- Supply Chain and Operations Analytics: Data analytics is applied in supply chain management and operations to optimize inventory management, logistics, demand forecasting, and production planning.
- Challenges and Considerations:
- Data Quality: Ensuring data quality and integrity is critical for accurate analysis and reliable insights.
- Data Privacy and Security: Protecting sensitive data and ensuring compliance with regulations such as GDPR and HIPAA is essential.
- Skill Gap: Addressing the shortage of skilled data analysts, data scientists, and data engineers is a challenge for organizations.
- Interpretability and Transparency: Making analytics models interpretable and transparent is important for understanding their decisions and building trust.
In summary, data analytics plays a crucial role in extracting actionable insights from data to drive informed decision-making, improve business processes, and enhance organizational performance across various domains. It encompasses a broad range of techniques, tools, and applications, with the potential to create significant value and competitive advantage for organizations that harness its power effectively.